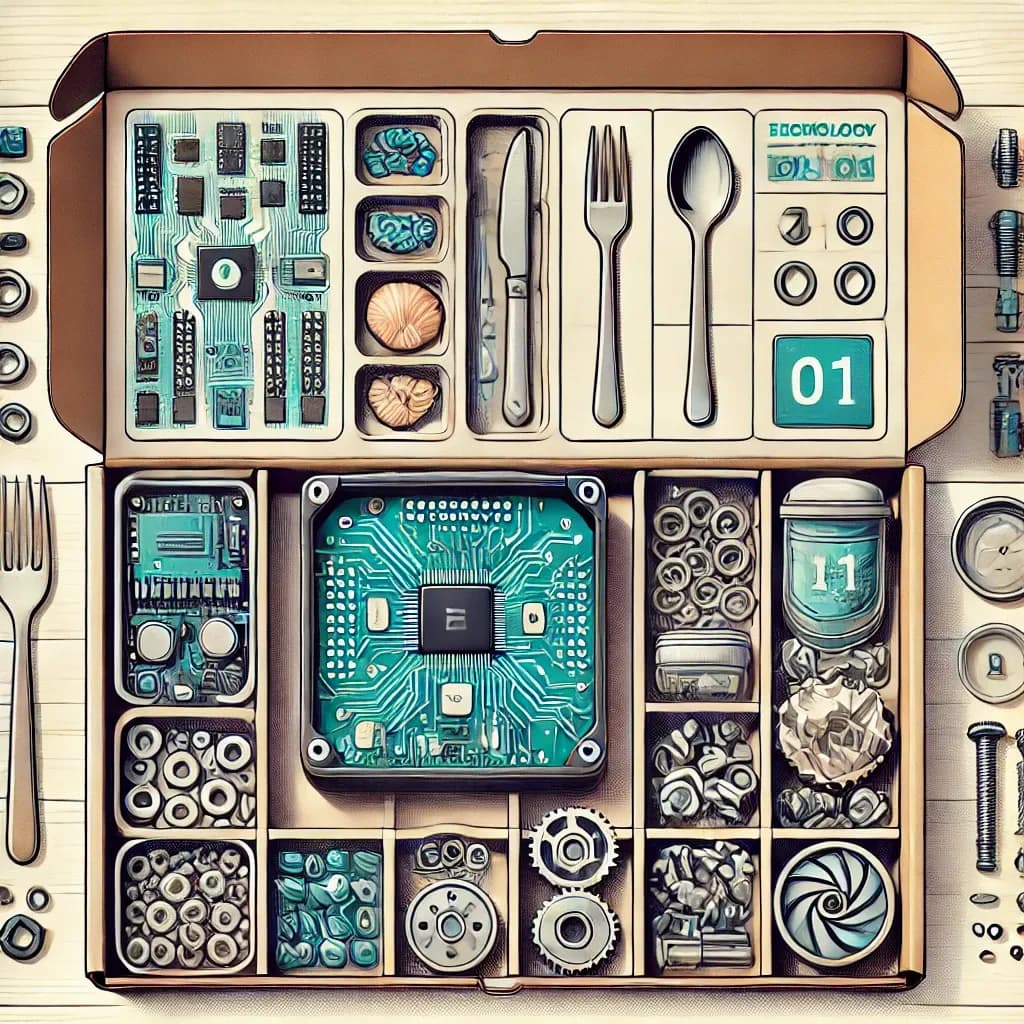
What’s critical for making informed technology investments is understanding where wrappers end and custom-built AI begins. GPT wrappers, or AI tools built on existing large language models (LLMs), have faced criticism in the market. Many assume these tools rely heavily on foundation models with minimal adaptation, adding limited value as a result. But most legal tech products are more than that.